Perovskite materials would be superior to silicon in PV cells, but manufacturing such cells at scale is a huge hurdle. Machine learning can help. — ScienceDaily
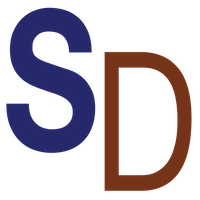
Perovskites are a spouse and children of materials that are at the moment the foremost contender to perhaps switch present-day silicon-based photo voltaic photovoltaics. They maintain the assure of panels that are far thinner and lighter, that could be produced with ultra-significant throughput at place temperature instead of at hundreds of degrees, and that are more affordable and simpler to transportation and install. But bringing these materials from controlled laboratory experiments into a merchandise that can be created competitively has been a long struggle.
Manufacturing perovskite-based solar cells requires optimizing at minimum a dozen or so variables at when, even within a single particular production tactic among lots of opportunities. But a new system based on a novel strategy to device learning could speed up the advancement of optimized production solutions and support make the subsequent technology of solar electric power a actuality.
The process, produced by researchers at MIT and Stanford University around the final couple of years, can make it achievable to integrate facts from prior experiments, and details based on private observations by experienced employees, into the device mastering approach. This tends to make the outcomes additional exact and has presently led to the producing of perovskite cells with an strength conversion efficiency of 18.5 percent, a aggressive stage for modern marketplace.
The exploration is noted in the journal Joule, in a paper by MIT professor of mechanical engineering Tonio Buonassisi, Stanford professor of supplies science and engineering Reinhold Dauskardt, new MIT exploration assistant Zhe Liu, Stanford doctoral graduate Nicholas Rolston, and 3 other folks.
Perovskites are a group of layered crystalline compounds defined by the configuration of the atoms in their crystal lattice. There are hundreds of this kind of attainable compounds and many various techniques of earning them. Though most lab-scale growth of perovskite elements works by using a spin-coating technique, which is not realistic for bigger-scale producing, so providers and labs all over the planet have been hunting for techniques of translating these lab elements into a practical, manufacturable product.
“You can find constantly a huge challenge when you happen to be hoping to just take a lab-scale process and then transfer it to something like a startup or a production line,” claims Rolston, who is now an assistant professor at Arizona Point out College. The staff seemed at a procedure that they felt experienced the greatest prospective, a process referred to as immediate spray plasma processing, or RSPP.
The manufacturing system would contain a relocating roll-to-roll surface area, or collection of sheets, on which the precursor remedies for the perovskite compound would be sprayed or ink-jetted as the sheet rolled by. The materials would then shift on to a curing stage, delivering a quick and continuous output “with throughputs that are greater than for any other photovoltaic technological know-how,” Rolston says.
“The authentic breakthrough with this platform is that it would allow us to scale in a way that no other product has allowed us to do,” he provides. “Even materials like silicon call for a significantly extended timeframe simply because of the processing which is performed. Whilst you can believe of [this approach as more] like spray painting.”
Inside that system, at minimum a dozen variables may impact the outcome, some of them more controllable than many others. These involve the composition of the starting up supplies, the temperature, the humidity, the velocity of the processing path, the distance of the nozzle utilized to spray the material onto a substrate, and the methods of curing the product. Quite a few of these variables can interact with each and every other, and if the procedure is in open air, then humidity, for example, may be uncontrolled. Analyzing all doable mixtures of these variables through experimentation is unachievable, so device studying was essential to help manual the experimental system.
But when most machine-finding out systems use raw data these types of as measurements of the electrical and other qualities of check samples, they really don’t ordinarily incorporate human experience these as qualitative observations produced by the experimenters of the visible and other houses of the examination samples, or data from other experiments claimed by other scientists. So, the staff located a way to integrate these exterior facts into the equipment discovering product, employing a probability component dependent on a mathematical system referred to as Bayesian Optimization.
Employing the process, he suggests, “possessing a design that comes from experimental information, we can uncover out traits that we weren’t equipped to see just before.” For illustration, they initially experienced hassle changing for uncontrolled versions in humidity in their ambient location. But the design showed them “that we could overcome our humidity difficulties by shifting the temperature, for instance, and by modifying some of the other knobs.”
The program now lets experimenters to considerably more speedily guidebook their system in order to improve it for a supplied set of ailments or needed outcomes. In their experiments, the group targeted on optimizing the electric power output, but the technique could also be made use of to at the same time include other criteria, these types of as price and longevity — anything customers of the staff are continuing to do the job on, Buonassisi claims.
The researchers were being inspired by the Section of Energy, which sponsored the operate, to commercialize the know-how, and they are at the moment focusing on tech transfer to present perovskite brands. “We are reaching out to companies now,” Buonassisi says, and the code they made has been manufactured freely out there by way of an open up-resource server. “It’s now on GitHub, anybody can down load it, anybody can run it,” he says. “We’re happy to enable firms get begun in making use of our code.”
Already, a number of companies are gearing up to deliver perovskite-centered photo voltaic panels, even even though they are nevertheless working out the particulars of how to deliver them, suggests Liu, who is now at the Northwestern Polytechnical College in Xi’an, China. He states businesses there are not however executing huge-scale production, but as a substitute commencing with smaller sized, large-worth purposes this sort of as making-integrated photo voltaic tiles where overall look is vital. A few of these organizations “are on track or are being pushed by buyers to manufacture 1 meter by 2-meter rectangular modules [comparable to today’s most common solar panels], in two yrs,” he claims.
‘The trouble is, they do not have a consensus on what manufacturing technological know-how to use,” Liu states. The RSPP technique, formulated at Stanford, “continue to has a good chance” to be aggressive, he states. And the device learning procedure the group designed could verify to be crucial in guiding the optimization of no matter what approach finishes up remaining applied.
“The primary intention was to accelerate the approach, so it necessary a lot less time, less experiments, and considerably less human several hours to acquire anything that is usable suitable away, for totally free, for field,” he states.
The team also integrated Austin Flick and Thomas Colburn at Stanford and Zekun Ren at the Singapore-MIT Alliance for Science and Technology (Intelligent). In addition to the Section of Energy, the operate was supported by a fellowship from the MIT Strength Initiative, the Graduate Investigation Fellowship Method from the Countrywide Science Basis, and the Clever software.